Data lakes: What they are, advantages, and considerations when designing one
Data lakes feed business intelligence systems. They're where data is collected, stored, and processed. Here are practices to consider when building a data lake.
Reading time: 3 minutes
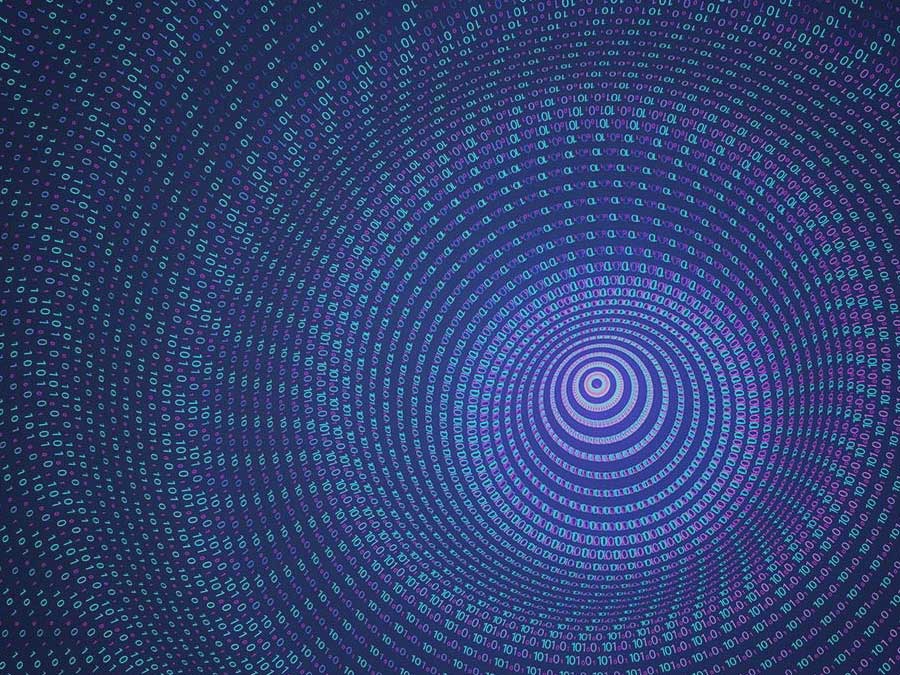
Data can be an enigma. While it can be a powerful tool and competitive advantage for businesses, simply having it does not create value. In fact, most businesses have more data than they realize yet few know how to access it…let alone activate it for actionable insight.
For most businesses, the prioritization of data is on point.
- Customers + (Data x Insights) = Customers2
The breakdown is in executing the equation. Customers and data alone do not equal insights. The data needs to be transformed and processed to create insights, which results in the actionable intelligence needed to inform business decisions. Data lakes serve as the central repository that feeds the process.
What is a data lake?
In simple terms, a data lake stores all the structured and unstructured data collected by an organization. The idea behind the data lake is to create a central repository that is easily accessible and usable for data analysis and processing.
Data lakes can be cloud-based or on-premise, but cloud-based data lakes are more widely adopted due to advantages with scalability and storage, as well as built-in data processing and analysis tools that simplify and bring cost efficiency to set-up.
The benefits of a data lake
Data lakes are valuable because they play a fundamental role in feeding business intelligence (BI) systems.
Traditionally, data would be warehoused. Prior to storing it, it would need to be processed and structured. This limited the types of data that could be processed and analyzed. Other data warehousing disadvantages include delays between collection and analysis, complexity of design and implementation, cost, and scalability.
By collecting, storing, and processing raw data, data lakes offer:
- Flexibility: any data type or format can be processed and analyzed on an as-needed basis by different departments across the organization.
- Scalability: easy to accommodate large volumes of data and expand to accommodate even more.
- Speed: processing is done as needed, leading to faster insights and decision making.
- Cost savings: typically offer the same efficiencies as other cloud-based solutions, including cost-effective storage options, security features, and built-in tools to simplify set-up and management.
- Data integration: by allowing for the collection, storage, and processing of raw data of different types and formats, the data lake can provide a unified view of an organization’s data. This allows for better insights and decision making.
Best practices when building a data lake
The concept of the data lake is great. It goes back to the formula shared earlier. The more customers an organization has the more data it has on its customers and the more it can learn from that data to earn even more customers.
Where data lakes fail (really, any data infrastructure system) is not knowing what you want to learn when designing it. Here are some best practices to consider when designing a data lake.
- Define the problem you want to solve. This will determine what data will be stored and the processes that will run alongside it.
- Choose a technology stack that fits the size and scale of both your existing technology infrastructure and your upcoming data lake needs.
- Implement proper data governance to account for data quality, security, privacy, and compliance.
- Establish a data ingestion pipeline. Pull data from various sources and develop the processes that will transform and clean it.
- Secure, monitor, and manage the data. Data should be encrypted and the data lake constantly observed to identify and resolve any issues.
Operationalize intelligence
When it comes to data, three common questions business leaders ask are: where is our data, what data do we have, and what can we learn from it?
Collecting, storing, and processing data in a data lake answers each question. More important, it makes the data accessible and easy to transform. The output is quick processing, ability to build predictive models, and reporting that can provide visibility into operations and insights into opportunities for improved performance and growth.